The overall goal of this project is to establish an innovative framework for nationwide smart precision aquaculture networks (SPAN), which will help farmers achieve sustainable shellfish production while preserving a healthy marine ecosystem.
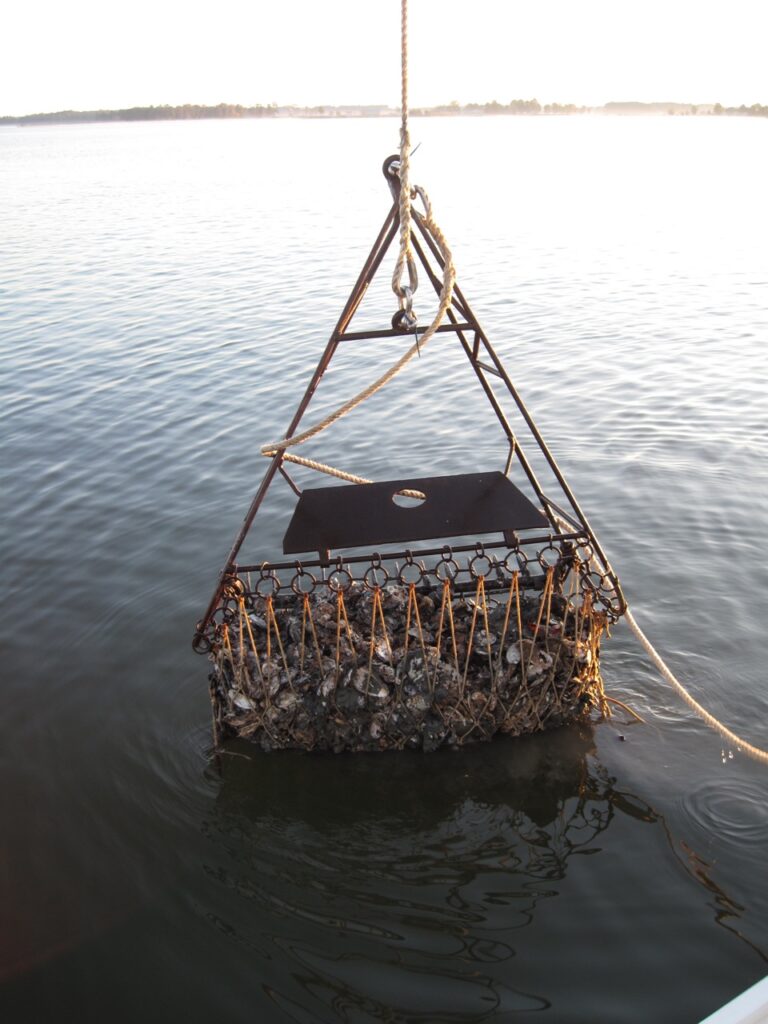
The Problem:
The current shellfish aquaculture industry is bottlenecked by outdated tools and technology.
The Solution:
We will develop precision tools to improve shellfish aquaculture production for global competitiveness and ecological sustainability.
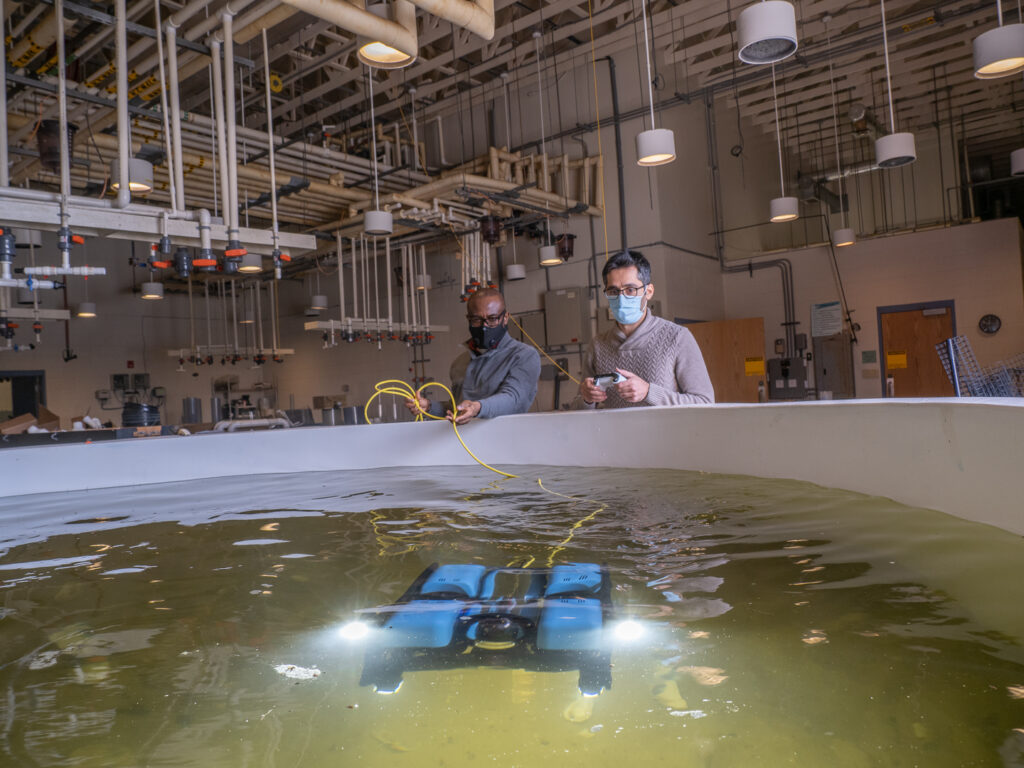
The U.S. has vast coastal areas suitable for significant aquaculture growth. However, domestic aquaculture is bottlenecked by outdated technology and tools. This is particularly true for on-bottom oyster farming, which has not changed over the past 200 years. This farming method involves random deployment of oyster seeds to the farm plot, which results in high mortality due to seed landing in unsuitable areas (e.g., suffocating in mud). After 2-4 years of growth, a towed dredge is used as the primary tool for harvesting. The grounds are blindly dredged twice: once for bringing up oyster clumps for manual separation (the separated oysters will be deposited back to allow further growth) and a second time for final harvesting. From planting to harvesting, the survival rate and production is extremely low.
Dredging activity can be environmentally damaging to oyster habitat and water quality, and has lethal and sub-lethal impacts on nearby oysters and other biota. In 2020, USDA NIFA funded a transdisciplinary team (involving 8 institutions along with industry partners from East, West, and Gulf coasts) led by PI Miao Yu through the Sustainable Agriculture Systems (SAS) program to tackle the challenges faced by the shellfish farming industry for achieving sustainable production. In the SAS project, underwater drone-based environmental and crop inventory monitoring are being developed to enable precision shellfish aquaculture on individual oyster farms, which paves the avenue for the proposed effort.
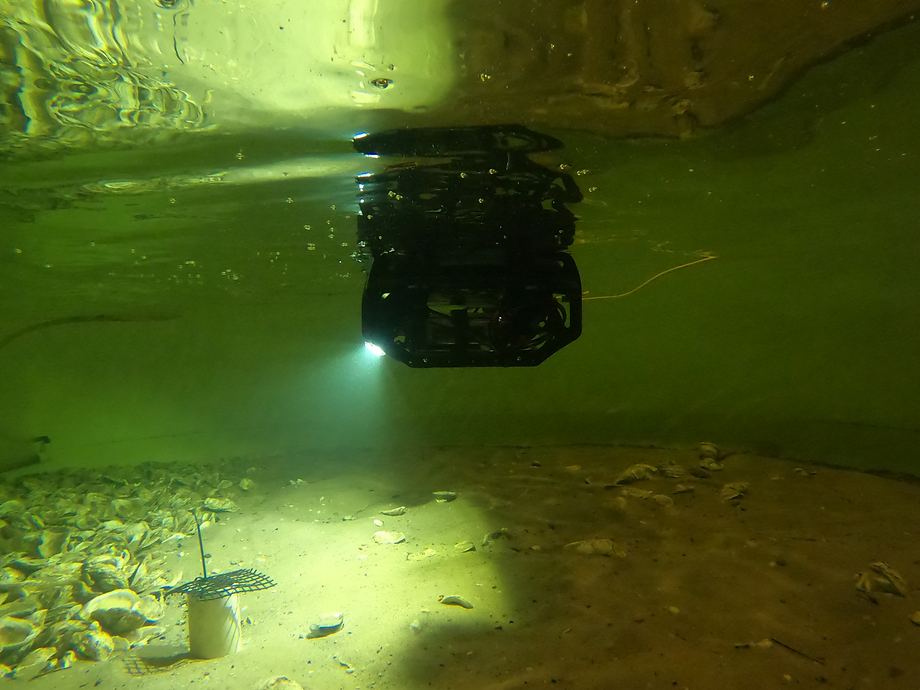
Marine Robot Control and Autonomy
Commercial robots (remotely operated vehicles (ROVs), autonomous surface vehicles (ASVs), and autonomous underwater vehicles (AUVs)) with host sensors hold great promise for survey tasks. Leveraging our progress on the USDA NIFA project, we will enhance the existing platforms with sensing and imaging capabilities. An ML classification, mapping, and re-routing pipeline for these robots to perform object identification and mapping in oyster farms will be developed by fusing different data types (e.g., camera, sonar). We will enhance the tethered single ROV operation developed in the USDA project with autonomous control and mobile sensor network operations.
The vast area of the Chesapeake Bay and its environmental variability makes it challenging to identify areas where oysters can thrive best. Site selection is a common problem in shellfish aquaculture, but has not received much needed attention. Poor site selection can result in low yield, ecosystem degradation, and poor relations among stakeholders and the general public. Currently, techniques that provide guidance for potential farm lease selection are not available. For the long-term expansion of the US shellfish aquaculture, identifying water bodies that support high productivity is crucial. Shellfish growth rate prediction within an existing lease is also critical for the optimization of shellfish production.
Predictive tools that interpret local environmental conditions to provide reasonable estimates of shellfish production would be of immense value to new and existing farmers. Models (e.g., FARM, ShellSIM, DEB) have been developed to predict production based on environmental, physiological, and production mode information. For example, the FARM model has been used to make yield predictions and to simulate production cycle, environmental effects, and economic optimization. However, the biggest obstacle to the use of these models is the availability of site-specific data for model parameterization and calibration to ensure accurate model output under heterogenous conditions.
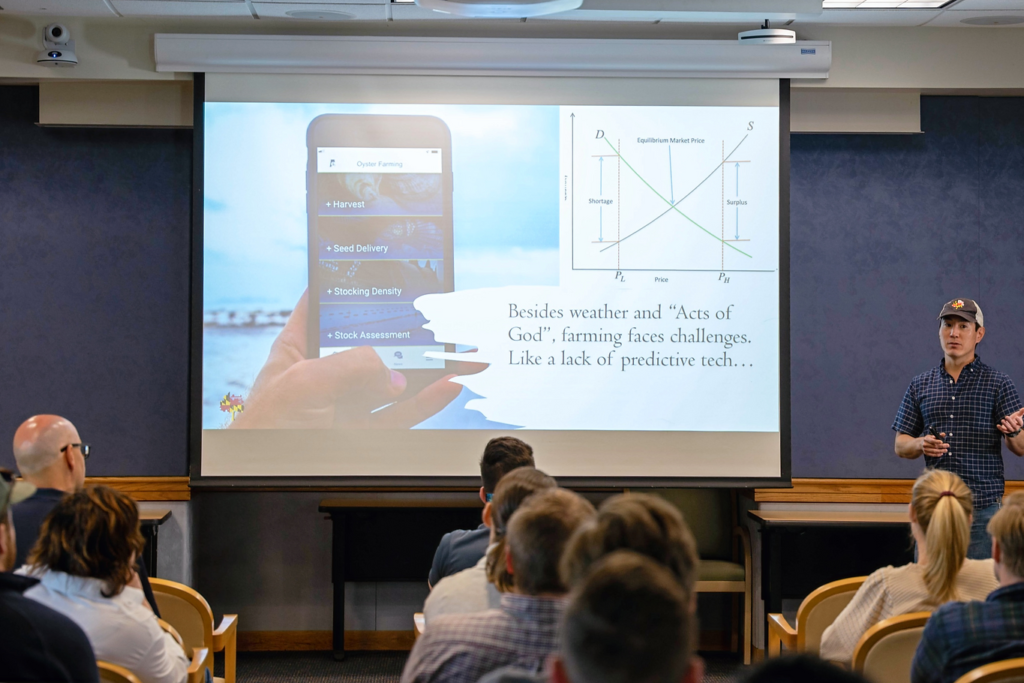
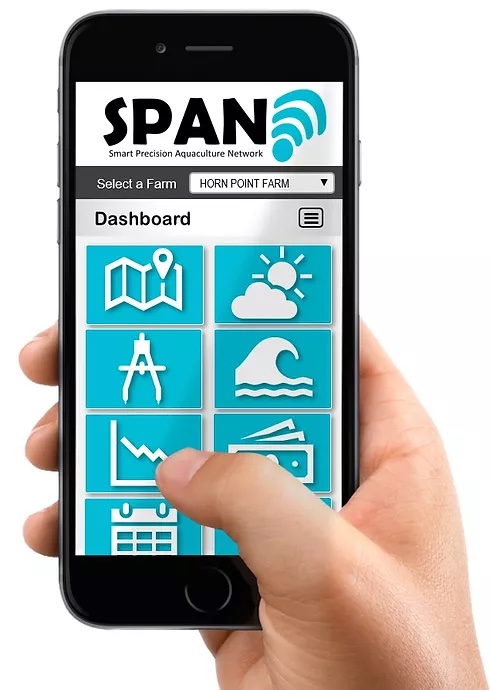
Smart Phone Application
Our partner institution, Fraunhofer USA Center for Experimental Software Engineering (CESE), will lead the app development, leveraging its deep competences in scalable software architecture, reliable AI systems, big data, advanced verification and validation, and user experience modeling. As the final SPAN product, the app will be designed to provide user-friendly data visualization and farm management solutions, based on the model outputs. We will work closely and iteratively with stakeholders to ensure model outputs are understandable and relevant. This app will help farmers reliably maintain their data assets, better manage and predict the volume, growth, and health of their shellfish crops, as well as develop and evaluate business plans for improving the economic viability for shellfish farming operations.
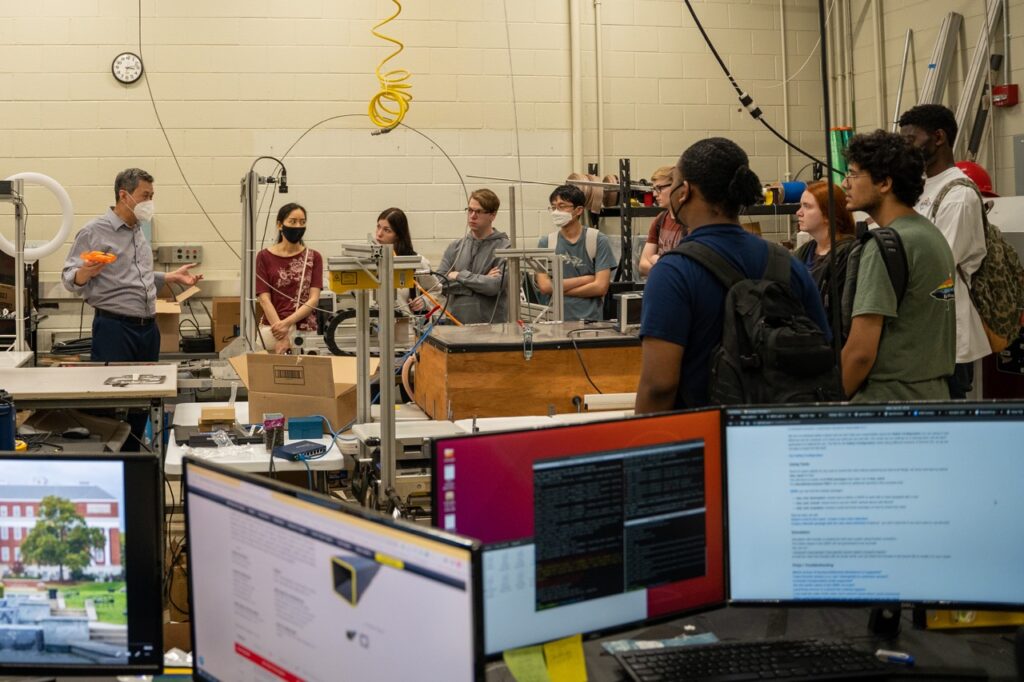
Broader Impacts.
SPAN education and training networks will be established to create education and training programs (e.g. research-driven program with social impact, user-inspired and societal need-based program) needed for promoting ecosystem sustainability. We will develop, implement, disseminate, and access new SPAN signature programs to prepare the future trans-disciplinary workforce to be globally competitive in science and technology innovation and social responsibility. These programs target 4 audiences: undergraduate students, graduate students, working professionals, and the general public.